Top AI Implementation Challenges and How to Overcome Them
Introduction to AI Implementation Challenges
Artificial Intelligence (AI) is revolutionizing industries across the globe, offering unprecedented opportunities for innovation and efficiency. However, implementing AI technologies is not without its challenges. Companies often face difficulties that can hinder successful integration and utilization of AI. Understanding these obstacles is crucial for businesses looking to leverage AI effectively.
Successfully implementing AI requires a strategic approach to navigate the common pitfalls and challenges. In this post, we will explore the top AI implementation challenges and provide insights into overcoming them.
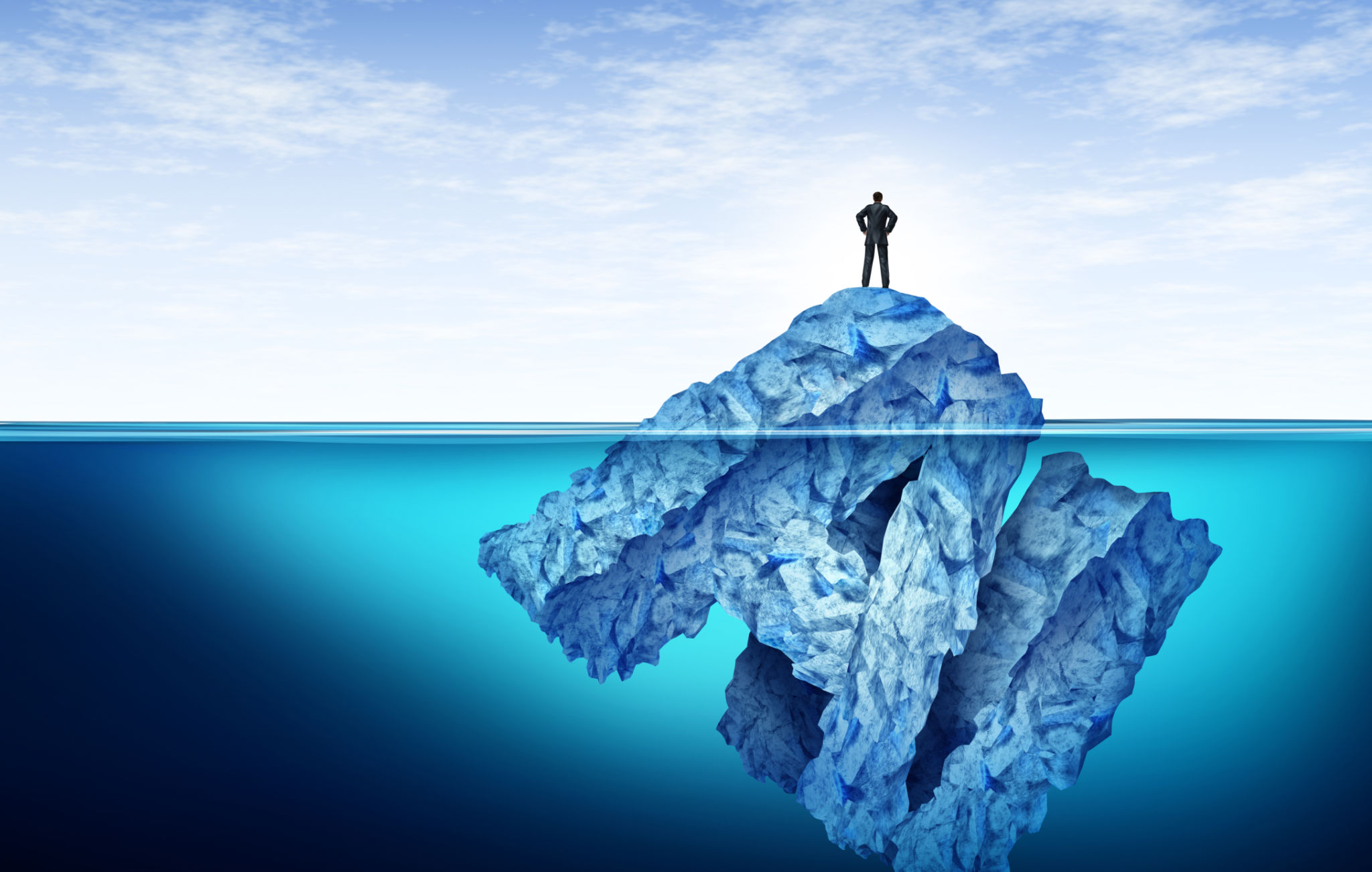
Data Availability and Quality
One of the primary challenges in AI implementation is the availability and quality of data. AI systems rely heavily on data to learn and make informed decisions. Without access to high-quality, relevant, and comprehensive datasets, the effectiveness of AI solutions is significantly compromised.
Overcoming Data Challenges
To overcome these data-related challenges, organizations should focus on data collection and management strategies. Implementing robust data governance frameworks and investing in data cleansing and preprocessing techniques are essential steps. Additionally, leveraging synthetic data or data augmentation methods can help in situations where real-world data is scarce.

Lack of Skilled Workforce
The shortage of skilled professionals is another major hurdle in AI implementation. The demand for data scientists, machine learning engineers, and AI specialists exceeds the current supply, leading to a competitive job market. This skills gap can delay projects and increase costs.
Building an AI-Ready Workforce
Organizations can address this challenge by investing in training and upskilling programs for their current employees. Collaborating with educational institutions to develop specialized courses or certifications can also be beneficial. Additionally, hiring external consultants or partnering with AI vendors can provide access to necessary expertise.
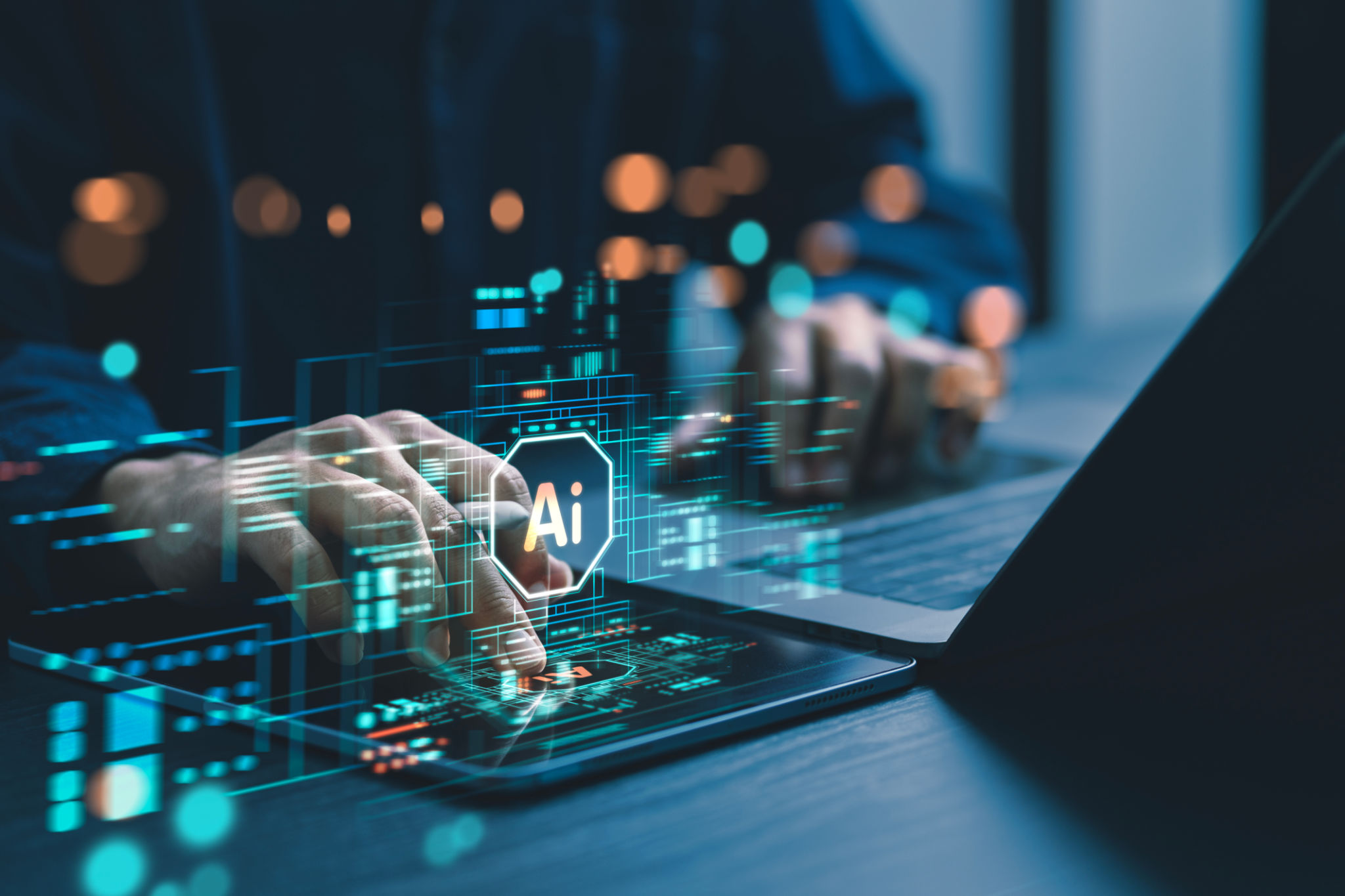
Integration with Existing Systems
Integrating AI into existing systems and workflows can be complex and time-consuming. Legacy systems may not be compatible with new AI technologies, leading to potential disruptions in operations.
Smooth Integration Strategies
To facilitate smooth integration, companies should conduct a thorough assessment of their current infrastructure and identify areas that require upgrades or modifications. Adopting a phased integration approach, starting with pilot projects, can help in minimizing risks. It's also beneficial to work closely with IT teams to ensure seamless integration.
Ethical and Regulatory Concerns
AI technologies raise ethical and regulatory concerns related to privacy, bias, and accountability. Companies must navigate a complex landscape of regulations to ensure compliance while maintaining ethical standards in their AI applications.
Navigating Ethical Challenges
Implementing transparent AI practices and developing guidelines for ethical AI use are critical steps. Organizations should establish clear policies for data privacy and conduct regular audits to identify and mitigate potential biases in AI algorithms. Engaging with stakeholders, including regulators and customers, can also help in addressing these concerns effectively.
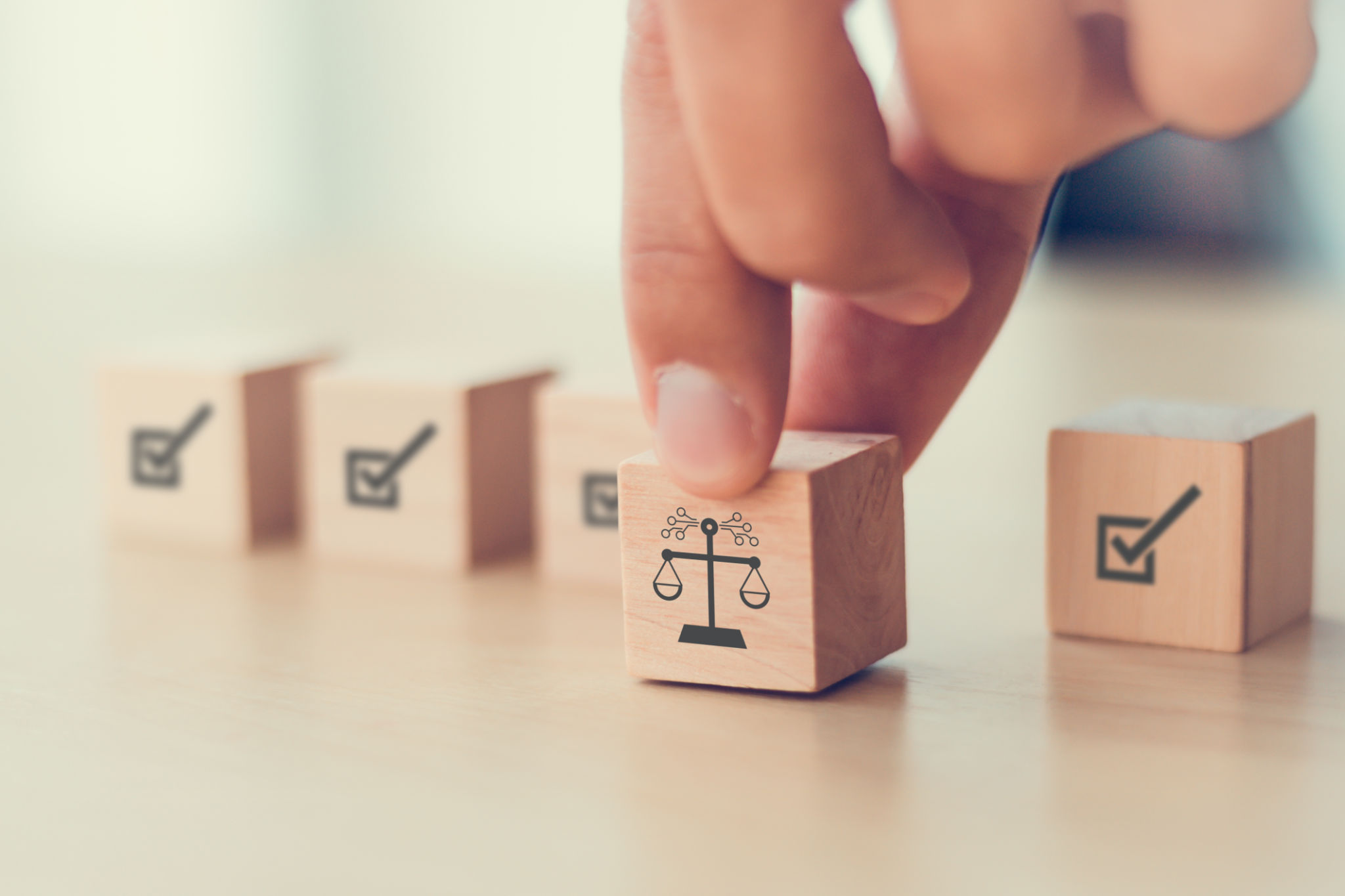
Conclusion
While the challenges of AI implementation can be daunting, they are not insurmountable. By understanding these obstacles and adopting strategic measures to address them, businesses can unlock the full potential of AI technologies. As the field of AI continues to evolve, staying informed about best practices and emerging solutions will be key to successful implementation.